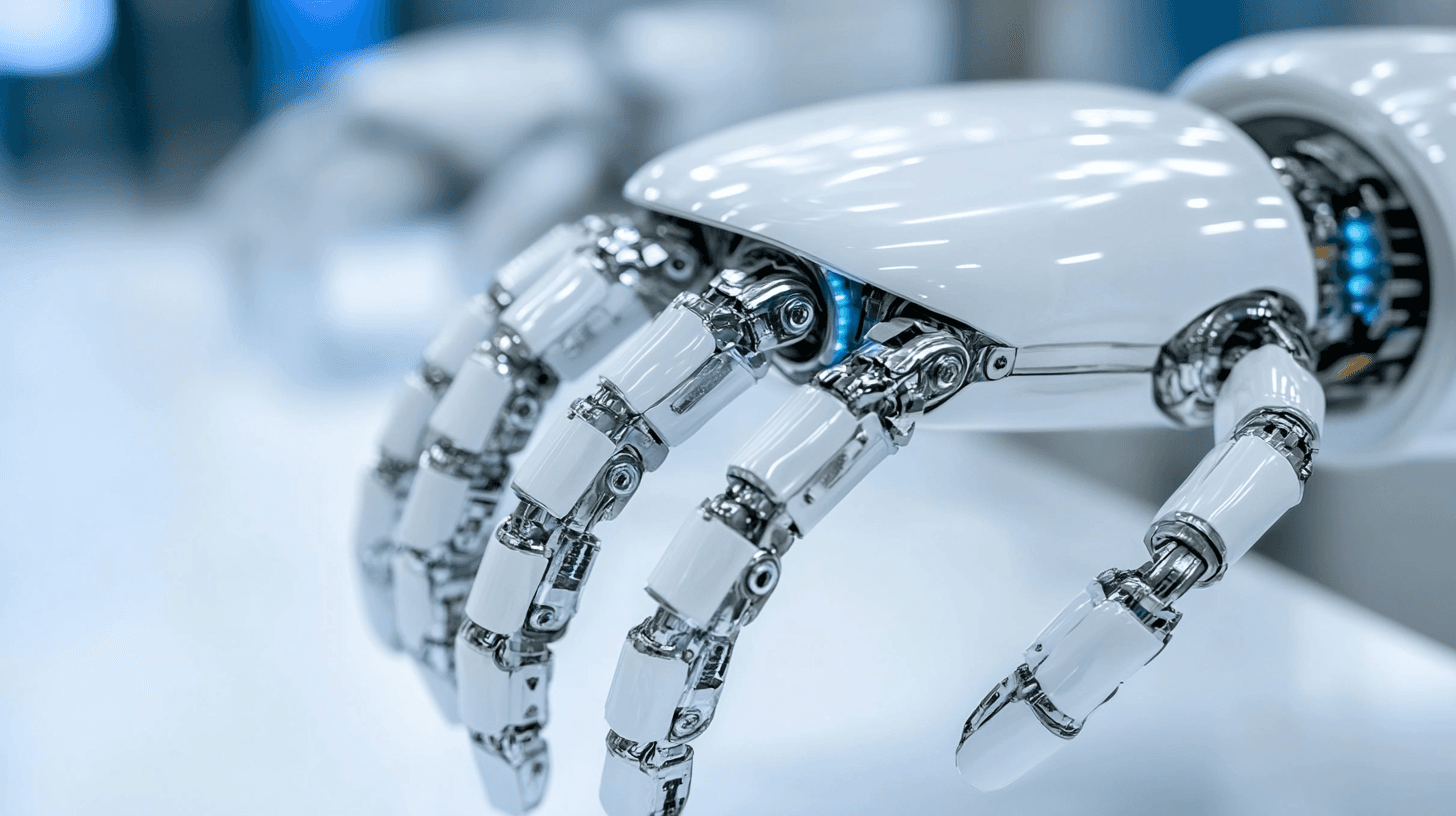
How AI and Machine Learning Are Transforming Cybersecurity in 2025
The cybersecurity landscape of 2025 bears little resemblance to that of even a few years ago. As we navigate through this year, artificial intelligence and machine learning have fundamentally reshaped how organizations approach digital security, creating both unprecedented defensive capabilities and alarming new threats. What was once considered futuristic technology has rapidly become essential for security professionals worldwide.
This transformation couldn't come at a more critical time. Today's threat actors are deploying increasingly sophisticated attacks at unprecedented scale, rendering traditional security measures woefully inadequate. The expanding digital footprint of modern organizations—cloud environments, IoT devices, remote workforces—has created an attack surface too vast and complex for conventional security approaches to protect effectively.
In this evolving digital battleground, AI and ML have emerged as game-changing technologies, enabling security teams to detect threats faster, respond more efficiently, and predict attacks before they materialize. But these same technologies are also empowering cybercriminals with new capabilities, creating what security experts characterize as a high-stakes arms race between defenders and attackers.
For tech leaders in businesses and government agencies navigating this complex security landscape, understanding how AI and ML are transforming cybersecurity isn't just academically interesting—it's strategically essential. Let's explore the key transformations, challenges, and opportunities in this rapidly evolving space.
The Evolving Cybersecurity Landscape
The integration of AI into security operations has accelerated dramatically in recent years, with adoption rates soaring across industries. Statistical projections indicate that by mid-2025, approximately 80% of large enterprises will have deployed AI-driven security platforms, a significant increase from just 50% in 2023.
This rapid adoption curve isn't merely following a technology trend—it's responding to measurable benefits. Research shows organizations implementing AI for threat detection have demonstrated a 35% reduction in breach incidents compared to those relying solely on traditional security measures.
The confidence in AI-powered security solutions remains high despite growing concerns about AI-enhanced threats. According to the Institute of Electrical and Electronics Engineers (IEEE), 44% of UK businesses remain convinced that AI applications will provide substantial advantages in the year ahead, particularly for real-time vulnerability identification and attack prevention.
AI-Powered Defensive Capabilities
Advanced Threat Detection
Unlike traditional security systems that rely primarily on predefined rules and signatures, AI and ML algorithms excel at analyzing vast quantities of data in real-time to identify patterns and anomalies that may indicate potential threats. The adaptive nature of these systems allows them to learn from historical data and evolve to recognize new attack methods, making them remarkably effective against novel threats that might bypass conventional security measures.
This capability is particularly valuable in environments where the volume of security alerts would overwhelm human analysts. AI systems can process billions of events daily, prioritizing genuine threats and reducing false positives that often lead to alert fatigue among security teams.
PERSONAL INSIGHT:
At Rezoud Inc., our implementation of AI-driven threat detection systems has reduced false positives by nearly 60%, allowing our security analysts to focus on genuine threats rather than chasing phantom alerts. This efficiency gain has dramatically improved our response times while reducing analyst burnout.
Automated Incident Response
AI-driven systems can now isolate affected systems, block malicious traffic, and implement predefined countermeasures without human intervention, significantly reducing response times and minimizing potential damage. This automation allows security teams to focus on more complex strategic issues rather than being overwhelmed by routine threat management.
The value of automated response capabilities becomes particularly evident during large-scale attacks, where minutes can make the difference between containing a breach and suffering catastrophic data loss. By streamlining the incident response process, organizations can maintain more robust security postures even as attack frequencies increase.
Behavioral Analysis and User Monitoring
Advanced AI systems now monitor user behavior patterns and flag deviations that may indicate compromised accounts or insider threats. This approach enhances security by focusing on real-time activities rather than relying exclusively on static authentication methods.
By establishing baseline behaviors for users and systems, AI can identify subtle anomalies that might indicate compromise long before traditional security measures would detect a problem. This capability is particularly valuable for identifying sophisticated attacks that might otherwise remain undetected for extended periods.
Predictive Analytics and Threat Intelligence
Perhaps the most transformative capability of AI in cybersecurity is its ability to predict potential attacks before they occur. AI systems analyze global threat feeds to identify emerging attack trends and anticipate potential threats before they materialize. This predictive capability enables organizations to strengthen defenses proactively rather than merely responding to attacks after they occur.
By leveraging machine learning to forecast potential vulnerabilities based on historical data and emerging trends, security teams can implement preventative measures that significantly reduce organizational risk profiles. This shift from reactive to proactive security represents one of the most fundamental transformations enabled by AI.
The Dark Side: AI-Enhanced Cyber Threats
While AI offers powerful defensive capabilities, it simultaneously enables more sophisticated attack methodologies. Cybercriminals have enthusiastically adopted AI tools to enhance their operations, creating increasingly challenging threats for organizations to counter.
AI-Powered Attack Statistics
The rise of AI-powered attacks has been dramatic, with research indicating a 67% increase in 2023 alone. By mid-2025, security experts project that cybercriminals using AI tools will orchestrate approximately 45% of all cyber attacks, a substantial increase from 30% in 2023. This rapid growth demonstrates the accessibility and effectiveness of AI-enhanced attack methodologies.
Sophisticated Phishing and Social Engineering
Cybercriminals now leverage machine learning algorithms to analyze vast amounts of data and create highly personalized communications that convincingly mimic legitimate sources. In 2025, advanced AI tools can generate entire conversation threads with appropriate context and references, making deception increasingly difficult to detect even for vigilant users.
The automation of phishing campaigns has made these attacks both more numerous and more effective, with research showing that AI-generated phishing attempts achieve success rates comparable to human-crafted approaches while requiring minimal resource investment from attackers.
Automated Vulnerability Scanning
AI has revolutionized vulnerability scanning, allowing attackers to identify system weaknesses at unprecedented scale and speed. By employing algorithms that continuously probe for potential entry points, hackers can discover and exploit flaws faster than organizations can patch them through traditional means.
This capability forces security teams to maintain constant vigilance and implement equally advanced defensive measures to keep pace with evolving threats. The automation of vulnerability discovery has significantly lowered the barrier to entry for potential attackers while increasing the operational burden on defensive teams.
Deepfake Threats
Perhaps the most concerning development in AI-enhanced threats is the rise of deepfake technology. Advanced AI systems now enable cybercriminals to create remarkably convincing audio and video impersonations of trusted individuals, facilitating highly effective social engineering attacks.
The implications are profound – an attacker might generate a video call appearing to come from a CEO requesting sensitive information or authorizing financial transfers, creating nearly undetectable fraud scenarios. As this technology continues to improve, organizations face increasing challenges in verifying digital communications and maintaining trust in electronic interactions.
PERSONAL INSIGHT:
Our security team at Rezoud Inc. recently identified and neutralized an advanced deepfake attack targeting one of our financial services clients. The attackers used AI-generated voice synthesis that mimicked the CFO so convincingly that it initially passed our standard verification protocols. This experience prompted us to develop enhanced multi-factor authentication that integrates behavioral biometrics and contextual analysis.
Emerging Technologies and Approaches
Adaptive Security Systems
Adaptive security systems leveraging reinforcement learning have emerged as a promising frontier in cybersecurity defense. These systems continuously learn from new threats and evolve their responses to counteract increasingly sophisticated cyber-attacks. Unlike traditional security systems with static defenses, adaptive frameworks modify their approaches based on observed attack patterns and outcomes, creating more resilient protection.
This adaptability is crucial in an environment where threat actors constantly innovate their techniques to bypass established security measures. By implementing systems that evolve alongside threats, organizations can maintain effective defenses even as attack methodologies advance.
Privacy-Preserving Machine Learning
One of the fundamental tensions in cybersecurity is the need to analyze sensitive data while maintaining privacy protections. Technologies like homomorphic encryption and federated learning ensure that sensitive information used for security purposes remains protected even during analysis.
These approaches allow security systems to identify threats without exposing the underlying data, addressing both security requirements and privacy concerns simultaneously. As regulatory requirements around data protection continue to increase, these technologies become increasingly essential.
Quantum Machine Learning
Quantum machine learning represents an emerging frontier with enormous potential impact on cybersecurity. This field leverages quantum computing principles to solve complex machine learning problems at speeds far exceeding classical computing capabilities.
The applications for cybersecurity are potentially revolutionary, particularly in areas like cryptography, threat detection algorithms, and complex pattern recognition. While still developing, quantum machine learning may fundamentally alter the security landscape by enabling computational approaches previously considered impractical.
Human-AI Collaboration
Despite technological advances, the integration of human expertise with AI systems remains essential. Security professionals increasingly recognize that the most effective defense strategies combine AI automation with human oversight to ensure systems remain effective and adaptive.
While AI excels at pattern recognition and processing vast data volumes, human analysts provide contextual understanding, strategic thinking, and ethical judgment that algorithms cannot replicate. This collaborative approach leverages the complementary strengths of both human and artificial intelligence to create more robust security frameworks than either could achieve independently.
Implementing Effective AI-Enhanced Security Strategies
Organizations seeking to leverage AI for cybersecurity must develop comprehensive implementation strategies that address both technological and human factors. While AI offers powerful capabilities, successful deployment requires careful planning, appropriate infrastructure, and ongoing management.
Framework-Based Implementation
Security frameworks like SOC 2 and NIST provide valuable guidelines for integrating AI while maintaining compliance with industry standards. These frameworks ensure that AI implementation follows established security principles while accommodating the unique characteristics of machine learning systems.
Risk Assessment and Tailored Approaches
Organizations must evaluate their specific threat landscape to identify vulnerabilities and determine where AI can provide the greatest security benefits. This tailored approach ensures that AI investments address actual organizational needs rather than following generic implementation patterns.
Different organizations face different threat profiles based on their industry, size, data types, and existing security infrastructure – effective AI implementation acknowledges these differences and adapts accordingly.
The Human Element
Despite technological advances, organizations must prioritize training and awareness programs to empower employees to recognize and respond effectively to emerging AI-enhanced threats. Building a security-conscious culture significantly reduces vulnerability to social engineering attacks, regardless of their technological sophistication.
Even the most advanced AI security systems cannot fully compensate for human error or negligence, making ongoing education an essential component of comprehensive security strategies.
Protecting AI Systems from Attack
Model poisoning represents an emerging threat specifically targeting AI security systems. Attackers may manipulate training data to introduce security loopholes that compromise AI effectiveness. This concern highlights the importance of data validation and protection throughout the AI lifecycle.
Organizations must implement rigorous safeguards for training data and regularly test systems for unexpected behaviors that might indicate compromise. The potential for AI systems themselves to become attack vectors adds another layer of complexity to security planning.
PERSONAL INSIGHT:
At Rezoud Inc., we've developed a dual-validation approach for our AI security models. Every model undergoes both automated adversarial testing and human red team evaluations before deployment. This combined approach has identified potential vulnerabilities that either method alone would have missed, significantly improving the resilience of our AI security systems against manipulation attempts.
Balancing Opportunities and Challenges
The integration of AI and ML into cybersecurity represents both unprecedented opportunity and significant challenge for organizations in 2025. These technologies have fundamentally transformed security operations, enabling more effective threat detection, automated response capabilities, and predictive defense strategies that were previously impossible.
However, they have simultaneously empowered attackers with sophisticated new tools for bypassing traditional security measures, creating an accelerating technological arms race between defenders and threat actors.
Key Considerations for Implementation
When implementing AI-enhanced security, organizations should consider:
1. Data Quality: AI systems are only as effective as the data they're trained on. Ensuring clean, comprehensive security data is essential for accurate threat detection.
2. Ethical Use: Implementing appropriate privacy safeguards and ensuring AI security measures don't create unintended biases or discrimination.
3. Integration Challenges: AI security tools must integrate effectively with existing security infrastructure rather than creating isolated security silos.
4. Talent Development: Building teams with both security expertise and AI understanding is critical for effective implementation and management.
5. Regulatory Compliance: Ensuring AI security measures align with relevant data protection and privacy regulations, particularly in highly regulated industries.
The Future of AI in Cybersecurity
As we look beyond 2025, several trends are likely to shape the future of AI in cybersecurity:
Autonomous Security Systems
The progression toward increasingly autonomous security systems will continue as AI capabilities mature. While human oversight remains essential today, future systems will likely handle more complex security decisions independently, particularly in scenarios where speed is critical. These systems won't merely detect and alert—they'll identify threats, contain them, implement countermeasures, and adapt defenses without human intervention.
The challenge will be balancing this autonomy with appropriate human governance to ensure security actions remain aligned with organizational objectives and ethical considerations. Organizations that successfully navigate this balance will gain significant advantages in the rapidly evolving threat landscape.
Democratization of AI Security Tools
As AI security technologies mature, we're seeing increased accessibility for organizations of all sizes. What once required specialized expertise and massive computing resources is becoming available through managed services and cloud-based platforms that reduce both technical barriers and cost considerations.
This democratization will help level the playing field between large enterprises with substantial security resources and smaller organizations previously disadvantaged by limited security capabilities. Cloud-based AI security services will continue expanding, providing sophisticated protection without requiring significant in-house expertise.
PERSONAL INSIGHT:
At Rezoud Inc., we've focused on creating scalable AI security solutions specifically designed for mid-market organizations that previously couldn't access enterprise-grade security. Our cloud-based offerings have enabled these clients to achieve security postures comparable to those of much larger organizations, demonstrating how AI can level the cybersecurity playing field.
Zero-Trust Architecture Integration
The integration of AI with zero-trust security architectures will continue accelerating, creating more dynamic and responsive security frameworks. Traditional perimeter-based security approaches have proven inadequate in modern environments with distributed resources and remote workforces.
AI enhances zero-trust implementations by continuously evaluating access requests based on contextual factors, user behavior patterns, device characteristics, and threat intelligence. This dynamic approach enables organizations to maintain robust security while minimizing user friction—a critical balance in effective security design.
Expanding Threat Surface Considerations
As organizations continue digital transformation initiatives, the cybersecurity threat surface continues expanding dramatically. IoT devices, operational technology, cloud environments, and remote workforces all create new security challenges that traditional approaches cannot adequately address.
AI-powered security will increasingly focus on managing this expanded threat surface through comprehensive visibility, continuous monitoring, and coordinated defense mechanisms. The ability to correlate security data across disparate systems and environments will become an essential capability rather than a luxury.
Government and Regulatory Implications
The integration of AI into cybersecurity has significant implications for government agencies and regulatory frameworks. Several key developments are shaping this landscape:
Enhanced Government Defense Capabilities
Government agencies face uniquely challenging security environments, combining highly sensitive data with sophisticated threat actors and extensive attack surfaces. AI technologies are revolutionizing government security operations by enabling more effective threat detection, improving incident response capabilities, and enhancing intelligence analysis.
Particularly for defense and intelligence organizations, AI-enhanced security provides critical advantages in protecting national security interests against state-sponsored threats and advanced persistent threats (APTs) that target government systems. The ability to process massive data volumes and identify subtle attack patterns has proven particularly valuable in these high-stakes environments.
Regulatory Evolution
Regulatory frameworks are evolving to address both the opportunities and challenges of AI in cybersecurity. Organizations should expect increasing regulatory focus on:
- Requirements for AI system explainability in security applications
- Standards for security AI model validation and testing
- Guidelines for privacy considerations in AI-powered monitoring
- Reporting requirements for AI-related security incidents
Forward-thinking organizations are proactively adopting standards and practices that anticipate these regulatory developments rather than merely reacting to them after implementation.
Public-Private Collaboration
The complexity of AI-enhanced threats has accelerated public-private collaboration in cybersecurity. Government agencies increasingly recognize that effective defense requires coordination with private sector expertise and resources, particularly in critical infrastructure protection.
These partnerships facilitate threat intelligence sharing, coordinate incident response activities, and develop collective defense capabilities that benefit both government and private organizations. For technology leaders, engaging with these collaborative initiatives provides valuable insights while contributing to broader security objectives.
Conclusion: Strategic Imperatives for Technology Leaders
The integration of AI and machine learning into cybersecurity represents both unprecedented opportunity and significant challenge for technology leaders in businesses and government agencies. As we navigate through 2025, several strategic imperatives emerge:
1. Adopt a Balanced Implementation Approach
While AI offers powerful security capabilities, effective implementation requires a balanced approach that:
- Integrates AI-powered tools with existing security frameworks
- Maintains appropriate human oversight and intervention capabilities
- Addresses both technological and organizational readiness factors
- Considers ethical implications alongside security objectives
Organizations that view AI security as merely a technological implementation rather than a comprehensive transformation often fail to realize the full potential of these technologies.
2. Invest in Security Data Infrastructure
The effectiveness of AI security tools depends fundamentally on the quality and accessibility of security data. Organizations should prioritize:
- Comprehensive security monitoring across all environments
- Centralized security data architecture with appropriate governance
- Data preparation capabilities that ensure AI models receive clean, relevant data
- Privacy-preserving techniques that enable analysis while protecting sensitive information
Without this foundation, even the most sophisticated AI security tools will deliver limited value.
3. Develop AI-Security Talent
The intersection of cybersecurity and artificial intelligence requires specialized expertise that remains in short supply. Forward-thinking organizations are addressing this gap through:
- Targeted recruitment of specialists with both security and AI backgrounds
- Upskilling existing security teams with AI knowledge
- Creating collaborative teams that combine security and data science expertise
- Partnering with specialized service providers to supplement internal capabilities
This talent development strategy is often the determining factor in successful AI security implementations.
4. Establish Governance Frameworks
As AI takes an increasingly central role in security operations, appropriate governance becomes essential. Effective frameworks should address:
- Accountability for AI-driven security decisions
- Transparency requirements for critical security processes
- Validation procedures for AI models before deployment
- Continuous evaluation of AI system performance and reliability
These governance mechanisms ensure that AI security systems remain aligned with organizational objectives and ethical considerations.
5. Prepare for Adversarial AI
Perhaps most importantly, organizations must recognize that the AI security landscape represents an ongoing arms race with increasingly sophisticated adversaries. Effective preparation includes:
- Adversarial testing of security AI systems
- Continuous monitoring for emerging AI-enhanced threats
- Scenario planning for potential AI security failures
- Resilience mechanisms that maintain security even if AI systems are compromised
This forward-looking approach acknowledges the dynamic nature of the threat landscape while building appropriate safeguards.
For technology leaders navigating this complex environment, the integration of AI into cybersecurity represents not merely a technical challenge but a strategic imperative. Organizations that successfully leverage these technologies while addressing associated risks will gain significant advantages in an increasingly hostile digital landscape.
The future of cybersecurity is undeniably intertwined with artificial intelligence and machine learning. By understanding both the transformative potential and inherent challenges of these technologies, leaders can chart a course that enhances organizational security while preparing for the continuing evolution of the threat landscape.
Looking to enhance your cybersecurity with AI? Rezoud Inc. can help!
Phone: +1 (855) 7-REZOUD
Email: contact@rezoud.com